Where are the ethical AI products?
It's time to talk about AI offerings that credit and compensate the people they rely on
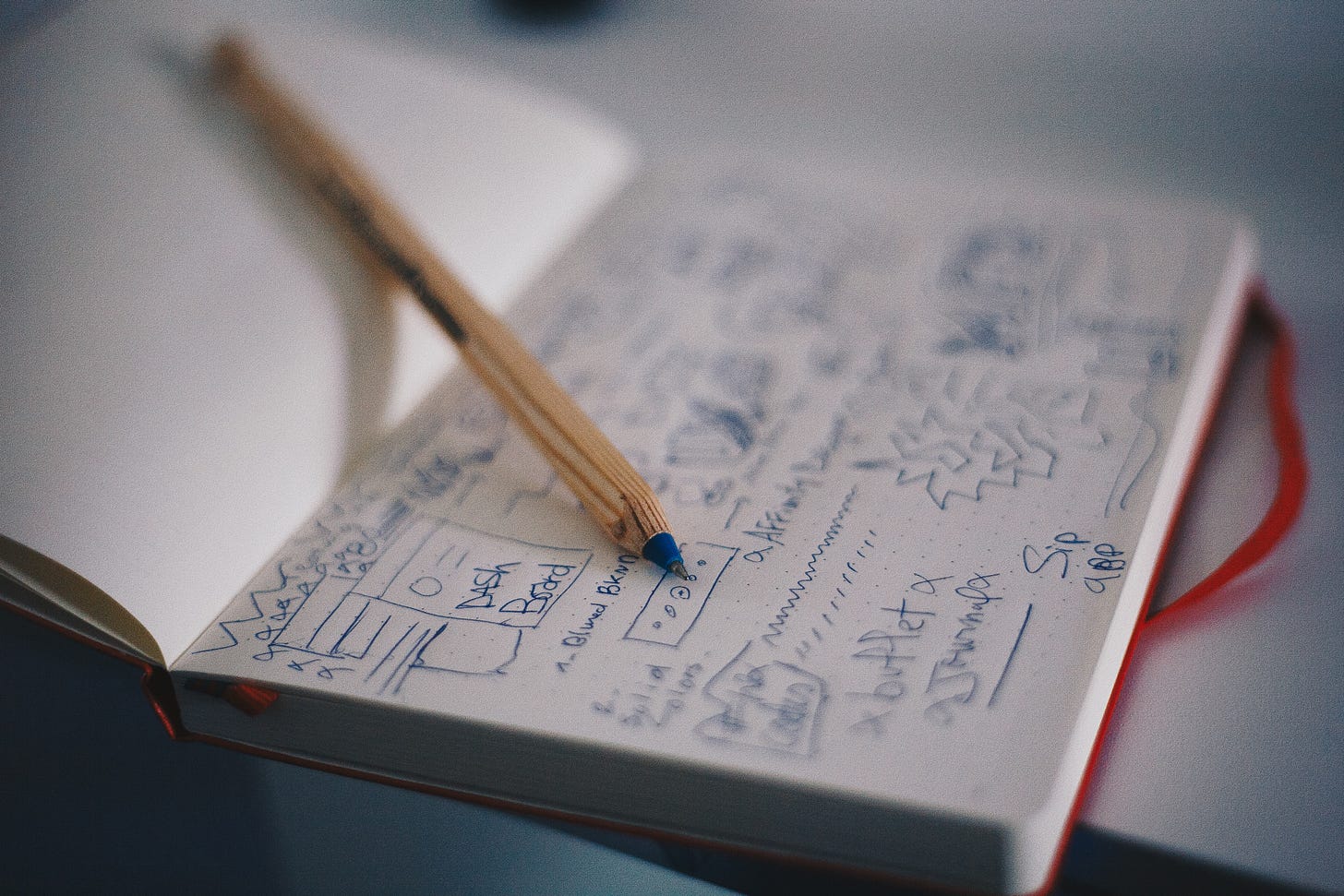
Artificial intelligence (AI), especially generative AI, has ignited discussions, debates, and controversies. While there are numerous benefits and applications of AI, it is essential to acknowledge and address the associated challenges. Generally, these challenges fall into three categories, popularized by Donald Rumsfeld:
Known Knowns: things we know we know
Known Unknowns: things we know we don't know
Unknown Unknowns: things we don't know we don't know
Known Problems with Generative AI
Known problems are discussed in various ways among different communities. In public discourse, they are relatively easy to talk about at a high level. The solutions to known problems tend to be less interesting to general audiences, but teams building products have to tackle them. For example, hallucinations, or the generation of responses that deviate from training data, are a known issue with large language models. In some contexts, such as for research applications, they are a known problem. In others, such as in generating art, they are a powerful capability. And, like many known issues with generative AI, they can be effectively mitigated and addressed through product development, design, and user guidance. So, the teams building generative AI products discuss known ways to modulate the degree to which AI responses include hallucinations and think through the design challenges and opportunities presented by them.
Generative AI for Art
Designers and developers can embrace or mitigate hallucinations when they focus their AI products on specific user needs. When using AI for visual art, hallucinations allow products to use the data they are trained on as a springboard for generating new creations. This can lead to visually impressive results while creating new challenges in recognizing the contributions of artists and other intellectual property rights holders. However, by leveraging existing models from industries that already deal with intellectual property rights, it should be possible to create equitable systems and business models that compensate artists appropriately. AI products have the potential to help artists protect their rights and monetize their work alongside creating new work.
For example, a product could integrate the byzantine licensing system for musical productions within a generative AI offering for music production. Imagine a product, let's call it Earmarker, that helps users generate new musical tracks and beats. It would rely on content from human musicians, much of it high quality but undervalued, to create music that has additional value while helping new audiences discover the work that was used by the system. A generative AI tool could help users with their creative work while tracking the use of source material so that artists who have opted in could receive compensation based on the use of their work, i.e., a stake of any revenue from the final musical production. Similarly, in domains like the visual arts and creative writing, AI systems have the potential to compensate and credit artists through commissions and royalties.
Known problems often overshadow unknown problems, which are more challenging to explore through conversations. However, thought leaders discussing AI ethics and safety bear the responsibility of directing their audience's attention thoughtfully because unknown problems pose the greatest risk due to being the last to receive attention. Addressing problems requires in-depth discussions, a strength of most academics, and attempts to solve them, a forte of business people. Integrating problematizing and problem-solving is necessary to create solutions that don't generate worse problems. Unfortunately, the current AI era reveals a disconnect between some industry experts and academics, as alluded to in our introductory skit. While polemic perspectives rarely help address problems, they can be engaging topics for conversation and content.
Some academics tend to dismiss the usefulness of generative AI, despite its widespread application across various industries, including academia, for increased productivity and real-world problem-solving. One reason for this disagreement may be the focus of some experts on how the technology works, while users concentrate more on the needs that AI products serve. The value of generative AI doesn't solely stem from the technology, which some experts downplay, but the wealth of high quality of data that’s available to generative AI systems, often freely, from people who’ve had their work appropriated by them. The tendency of generative AI products to decouple expertise and creativity from human knowledge and artistry is one of the major known problems with generative AI. However, there are known solutions that could usher in an era where people monetize their work through datasets for AI systems, or, in a bleaker alternate future, have their work taken without permission as they become obsolete.
In the realm of generative AI for artistry, hallucinations allow responses to deviate from their source material to create something new. By tracking the automated use of inputs, AI products for art can serve their purpose while addressing even more significant problems, such as the challenge many great artists face in making a living through their work. The intellectual property rights of artists were under threat long before ChatGPT and Midjourney. For example, some creators have found success by buying second-hand art and adding interesting elements, like a brand logo or celebrity photo, without facing the pushback that artists using generative AI receive. Integrating artists into the value chain of products relying on their data should be a no-brainer because it's both a business opportunity and an ethical imperative. And employing a business model that relies on appropriating art without the creators' consent is risky, as ongoing lawsuits have the potential to disrupt companies taking an "it's easier to ask for forgiveness than permission" approach. Grace Hopper's wise words don't apply when suing is a viable option.
Generative AI for Research
While generative AI holds creative potential valuable to the arts, its application in science and research poses challenges. Researchers require accurate data rather than phantom quotes and hallucinations from AI. Designers and developers can implement measures to address this issue, such as designing products to restrict responses to specific data sources, displaying citations, and facilitating users in verifying response accuracy through thoughtful features and clear communication.
AI systems, whether used for research or artistry, can compensate and credit individuals who have consented to the use of their work. While implementing equitable business models presents complexities in the arts, these issues are more straightforward in the context of research. Companies and institutions already invest billions in research, and generative AI can assist researchers across industries and academia in making better use of research reports, books, and interview transcripts. Furthermore, the authors of materials used for secondary research often make excellent interviewees. The integration of secondary research and expert interviews through AI is a unique aspect of Ferret, our AI chatbot that credits and compensates the individuals who contribute to it.
Expanding the Narrative
Generative AI has known problems that can be effectively addressed by integrating research, design, and tech ethics. The most discussed problems with general-purpose offerings like ChatGPT are often the easiest to tackle in more focused AI products. And while it may seem that generative AI exploits unwitting human contributors, that’s not the full story. As we work on developing Culture Capitalist into a resource for the tech community, alongside professionals from related industries and human science, we’ll highlight the work of people creating AI products designed to uplift and empower human contributors rather than erase them. We plan to publish follow-up reports and articles to assist innovators in developing more ethical business models. Additionally, by showcasing successful solutions to known problems, we aim to draw attention to issues that still lie in our blind spots—problems we genuinely cannot solve because they have yet to be discovered.
If you are building an AI product with an equitable business model or are interested in monetizing your expertise through interviews with people who value it, please use the button below to join our network or visit askferret.com.